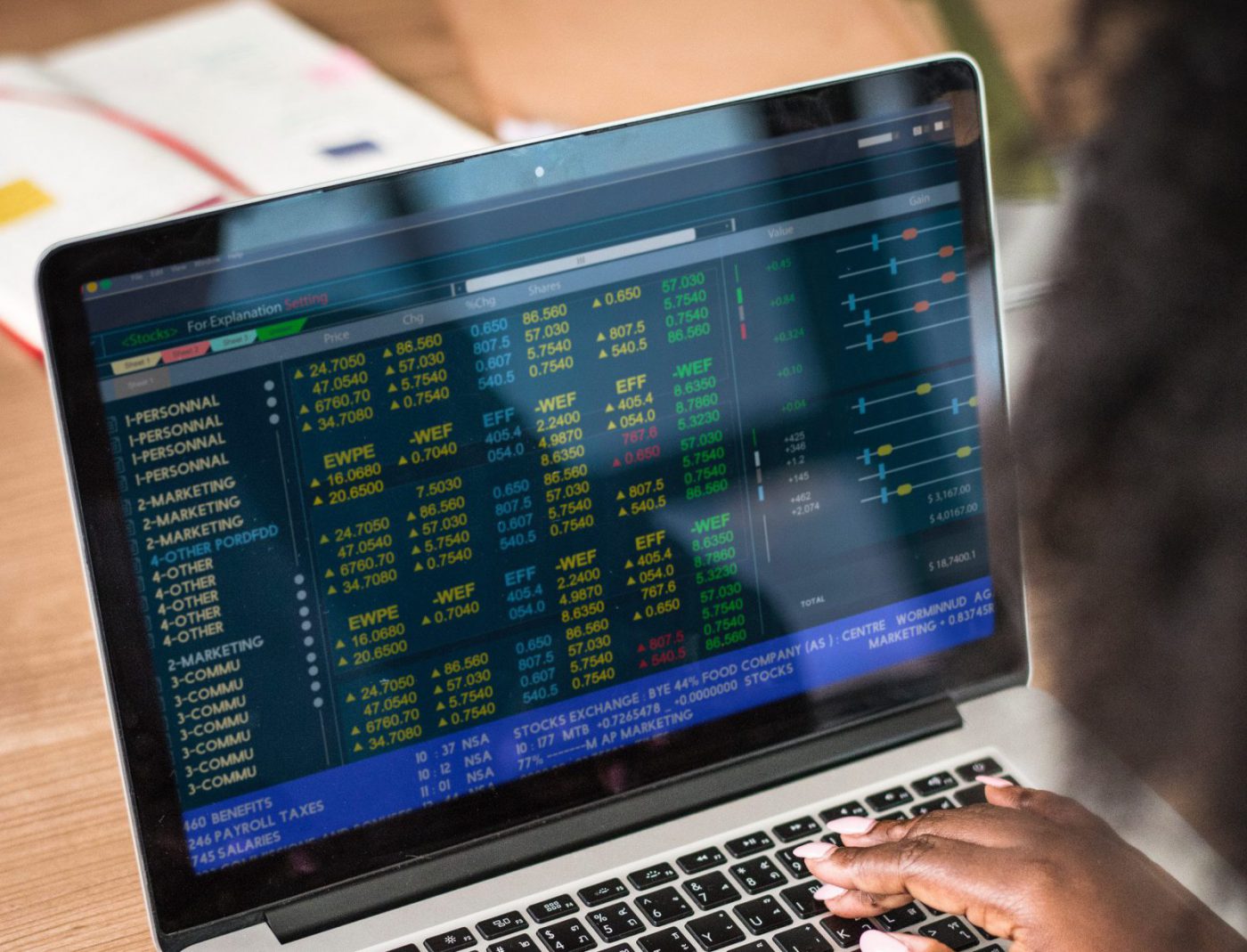
Can we identify a group of newborn children at high risk for future obesity?
Researchers are seeking to answer questions like this with the aid of machine learning, one of the hottest topics in the scientific community.
What is machine learning? It is a branch of artificial intelligence and a method applied to help computers make predictions or decisions based on the data they have received, or learned. In the United States today, we live surrounded by products made with this technique. Have you received advertising that seems to know your purchasing habits? Machine learning likely was involved.
In Arkansas, the state with the highest school-age obesity rate in the nation, we have identified childhood obesity as a characteristic that is unlikely to change over time. In a project ACHI conducted in collaboration with Arkansas Medicaid, we determined that 74% of students entering kindergarten at normal or obese weight status remain in that status by grade 8.
As we prepared policy recommendations based on this finding, we wondered: If one can predict future weight status with such high accuracy among kindergarteners, what is the earliest we can predict future weight status? Can we go back to birth? And if future obesity is “written in the stars” at birth, then what role does environment play in shaping children’s weight status? It turns out we can do a pretty good job of predicting obesity by looking at birth certificate records and environmental factors in the counties where the children reside.
ACHI’s machine learning prediction model, which my colleague Dr. Tony Goudie and I discussed at AcademyHealth’s Annual Research Meeting in Washington, D.C., in June, can predict newborn children’s kindergarten weight status with 83% accuracy. Also, 30% of newborn children identified to be at high risk by this model become obese by kindergarten. My presentation also touched on identification of factors that are predictive of obesity. Among environmental factors, free and reduced school lunch program enrollment, student-teacher ratio, and county-level rate of population with no leisure-time physical activities are all strong predictors. At the individual level, gender, race/ethnicity, birth weight, mother’s weight gain, mother’s age at birth, mother’s smoking status, mother’s marital status, number of prenatal doctor’s visits per month, birth order, and parents’ education are important variables.
We are wrapping up this project by fine-tuning the model and sharing our methodology with healthcare policy and machine learning communities. Keep an eye out for another machine learning blog post!